New Ingestible Device Can Remotely Monitor Vital Signs with Accuracy
The capsule-sized device can provide accurate measures of respiratory and heart rate, enabling sleep apnea monitoring, a human trial shows.
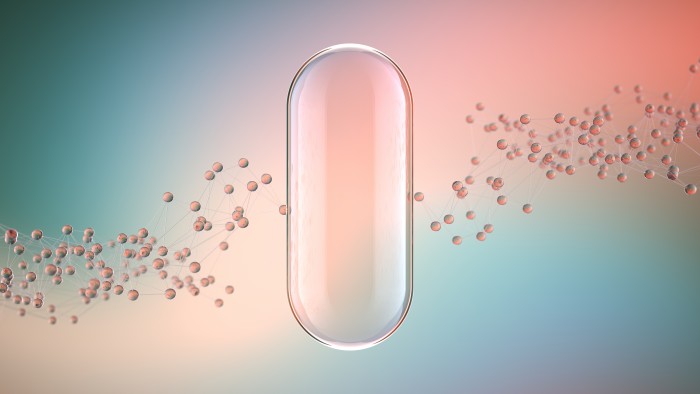
By Anuja Vaidya
– Massachusetts and West Virginia researchers have developed and evaluated a wireless ingestible device that can enable remote monitoring of vital signs.
The Vitals Monitoring Pill (VM Pill) is a capsule-size device that can accurately collect and report vital signs, including respiratory and heart rates. It leverages a custom configuration of integrated circuits and electronic sensors, including an accelerometer, to measure small ballistic movements generated in the gastrointestinal tract each time the heart beats or breathing occurs.
Researchers at Brigham and Women’s Hospital, Massachusetts Institute of Technology (MIT), Celero Systems, and West Virginia University developed the device and assessed its performance. First, they evaluated performance in a preclinical animal model study, which showed the device could detect signs of opioid-induced respiratory depression. They then conducted a human trial among patients in a sleep study, the results of which were published in the journal Device.
The research team included ten patients in the study, gathering 57 hours of patient data. These patients were scheduled for sleep study evaluation at West Virginia University (WVU) Medicine between December 2021 and August 2022. About 30 percent were diagnosed with central or obstructive sleep apnea (OSA) and wore a bilevel-positive airway pressure or continuous positive airway pressure device during the study.
The trial shows that the VM Pill was able to gather respiratory and heart rate data comparable to data collected from existing monitoring devices. On analyzing the data, researchers observed that the device captured respiratory rhythms within the expected range of 9 to 25 breaths per minute and cardiac signals within the scope of 40 to 95 beats per minute.
Further, the device pinpointed moments when subjects stopped breathing, either during sleep apnea or because they were intentionally holding their breath. The interference of the external sleep apnea monitoring device did not affect signals from the VM Pill.
“Our study provides a tangible product with real commercial value,” said study co-corresponding author Giovanni Traverso, MB, PhD, a gastroenterologist at Brigham and Women’s and associate professor of mechanical engineering at MIT, in the press release. “Ingestible vital monitors can really transform our capacity to rapidly respond to life-threatening events.”
Future development plans include incorporating technology that can keep the device in the stomach for a week and creating closed-loop systems that can detect apneic episodes and provide on-demand drug release to automatically detect and reverse an opioid overdose.
As mHealth technologies become more advanced, their use cases grow increasingly varied.
For instance, researchers from the Marcus Autism Center, a Children’s Healthcare of Atlanta subsidiary, recently developed a tablet-based diagnostic tool to diagnose autism spectrum disorder.
Children watch videos of social interaction on the portable tablet, which monitors their “looking behavior” to pinpoint the social information the children are looking at and the information they are not looking at, according to a press release.
Clinicians review the data collected by the device, including a personalized report with visualizations from the test. They can then provide pediatric patients and their families with a diagnosis and measures of the child’s individual abilities, including social disability, verbal ability, and non-verbal learning skills.
Wearable sensors can also help clinicians remotely measure gross and fine motor function in patients with amyotrophic lateral sclerosis (ALS).
Research from Massachusetts General Hospital and ALS Therapy Development Institute found that analyzing wearable sensor data provided “reliable, sensitive, and interpretable measures” of motor function in ALS patients. Researchers gathered accelerometer data from sensors worn on the wrists and ankles by 376 individuals with ALS.
They used features extracted from the data, like submovement and activity bout, to create two supervised machine-learning approaches to produce composite measures of overall motor impairment.